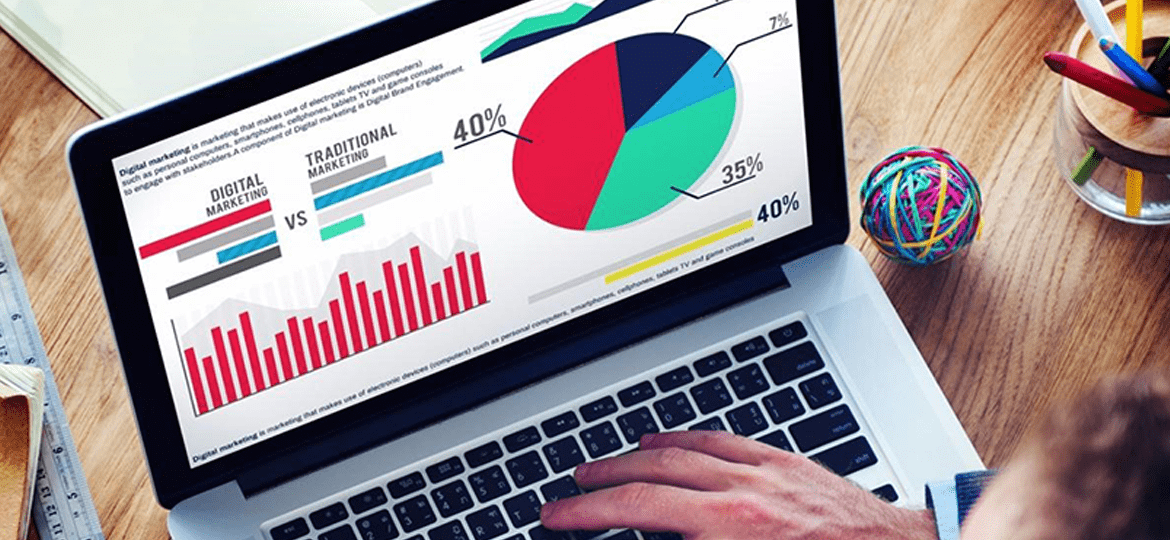
Marketing Response Model (MRM)
For many companies, marketing campaigns are the key to both communicating with their market, and for grabbing the customer’s attention. But it is not entirely possible or even advisable to target your entire customer base using just one campaign. The most obvious reason being the waste of whatever limited marketing budget allocated: sending out irrelevant campaigns to customers who wouldn’t even bat an eye.
To make matters worse, some customers might even get so exasperated, that they become averse towards your product or brand. Hence, the ideal marketing tactic would be to send out your marketing message only to those customers who would really appreciate it. The analytical solution to this is called Marketing Response Modelling- developing models that predict the likelihood of a customer to respond to a marketing offer.
Marketing Response Model
The aim is to develop a classification model that filters a list of customers who are likely to respond to your call for action. In other words, MRM focuses on deepening or recovering customer relationships using analytical models.
When developing an MRM, different types of responses should be considered. For instance, we could invest in an email marketing campaign or a Facebook Ad which has a fancy banner with a link redirecting to your website. We can now quantify the response in many ways. Viewing the ad could be the first type of response, a soft response, which is of importance since it will create an awareness of the product and/or the brand. The next response, a hard type, would be the person clicking the link. Click through shows that the offer has already generated a maximum engagement.
Responses may then come in a variety of forms. Opening a web page or downloading a pdf with product details, sharing contact details with a request for the price quote, or subscribing to a service to name a few. These are examples of soft responses that have piqued an interest in your ad/offer. But the final goal is to generate a hard response, i.e. product or service purchase. Hence, the first step in MRM would be the data scientist discussing the target (click through, pdf download, or forthright purchase) that needs to be modeled by the business expert.
Understanding Customer Responses
After defining a satisfactory target response, the historical data, which is the key for analytical modeling, need to be gathered from previous marketing campaigns. This is essential to properly understand customer responses. Popular examples of data that might be useful are:
- Demographic variables (e.g., age, gender, marital status, employment status)
- Relationship variables (e.g., length of relationship, number of products purchased)
- RFM variables (see above).
- Social network information (e.g., purchase behavior of friends, product reviews from friends)
All of the above data elements can then be gathered into a dataset to build your analytical response model.
Logistic regression, decision trees, neural networks and random forests are some of the analytical techniques commonly used. While logical techniques help us understand why customers respond, companies prefer to know who will respond. Hence, black box analytical models (e.g., random forests, neural networks) are the crowd favorite. Another approach to response modeling- the regression model can help in quantifying the response (amount/intensity).
Predictive Marketing Response Model
Here is an example of how predictive Marketing Response Models can be used for getting insights on customer responses to a pricing action. It does not take a genius to decipher that companies have to be competitive in their pricing strategies in order to remain relevant. But, is it that easy to predict the outcome of such a strategy?
Predictive analytics is the solution to the most challenging component of any analytically driven pricing strategy – estimating customer response. Using a Marketing Response Model (MRM) or the demand model, as it is otherwise known, we can estimate customer response.
The formula for constant elasticity model:
This model makes use of the concept of Elasticity.
“Elasticity is the percentage change in demand over the percentage change in price.” It is derived using a reference price.
A graph showing the three commonly used Market Response Model forms.
In an ideal case, best results are produced by examining experimentally controlled studies on original customer purchase data. Clinically controlled experiments and survey feedbacks are often unreliable. Looking at “Consumer purchasing behavior”, we can get a better idea of customer price response as it is the best data for gaining insights.
The biggest drawback of controlled “live customer behavior experiments” on market price is that they are difficult to automate. The risk of losing customer patronage if found selling at different prices to different customers is also high. The best alternative is relying on the company’s “historical transaction data”.
Market Response Models, in this case, are built using historical transaction data by leveraging data mining to mimic a “controlled price experiment” by defining the boundaries of the experiment namely lead-time, alternative offers and seasonality, then picking only observations that fit the criteria. Historical transactions of an organization usually contain millions of transactions, most of which are uncovered, that meet the exquisite requirements of a controlled price experiment. This huge sample size is an added advantage in estimating the Market Response Models, in a statistical sense that it increases the ability to detect a relationship to price, something where the smaller live market or laboratory experiments fail.
Marketing Response Models such as the one described above are easily repeatable, responsive to automation, and in the long run very inexpensive. They are the ideal marketing solution for a targeted marketing approach.